Understand your dataset quality through our newest addition: Dataset Quality Analytics
Safe AD or ADAS vehicles require an excellent perception system to be able to perceive the surroundings, interpret them and move accordingly in a safe way. The foundation of such systems often relies on deep neural networks which are trained on massive amounts of data. As you can imagine, developing these models requires a lot of work. You need a great infrastructure to run multiple experiments at the same time to increase your efficiency on how you improve your models. In addition, you need a lot of data engineering to populate those training runs with the right scenarios you want to train on.
In this line, we must say that our customers are very skilled doing these core jobs. When an issue in their perception systems arises, they usually are great at debugging their data pipelines and running experiments with adapted data augmentation methods or with different network architectures, to mitigate the issue. However, and more often than not, we have observed that perception system developers come with two frequent questions when debugging their models, finding themselves having a hard time when trying to answer them:
- Do I have sufficient volume of data to train this model on this scenario or class?
- Is the label quality sufficiently good to train this model on this scenario or class?
In this blogpost we will focus on giving an answer to the latter question. Here, our newest data quality product has a key role to play as it will help your teams see if they have the right data. And, yes, you guessed right: we won’t leave the first question unanswered. We will cover it in another blog post as part of our upcoming Data Coverage product release 👀. And now, let’s dive into today’s topic. 👇
Is the label quality sufficiently good to train this model on this scenario or class?
Let’s go back to the start: understanding if annotations are good enough is a struggle many companies face. What’s the recommended approach, if any?
Most people spend a lot of time browsing data trying to understand whether the overall structure is good enough or not. In the Kognic Data Quality team, we have seen that this work is often semi-structured, the result of it being that some data points are reported as mislabeled and they need to be fixed. Even though annotations are a manual process that always come with some errors, the truth is that, normally, there are more irregularities in the data due to different interpretations and the labeling guidelines which are hard to find by just browning data.
The key in all this is that you need objective dataset quality metrics to be able to argue if your dataset is good enough. Fortunately, we have the knowledge and the resources to help with that 😎. For some time now, we have been developing methods to measure dataset quality in a robust way, which has enabled us to identify structured errors and has already helped our clients to understand if they have the data they need. And, finally, we can proudly say that our work has borne fruit. Meet our newest product: Dataset Quality Analytics. ⚡
Dataset Quality Analytics. What’s in it for you?
In case you wondered, licensing our brand new Dataset Quality Analytics product is all perks.
First of all, by measuring dataset quality with our DQA you won’t need to browse massive amounts of data anymore to understand it: you will be able to easily navigate through your quality metrics and drill down on the ones you find suspicious to understand the origin of the issue. If when looking at those metrics you think they can be improved, the most effective way often is to clarify the guideline so annotators can understand it better.
Besides providing you with a sophisticated way to confirm that your data is good enough and helping you identify issues in your data, DQA will allow you to find out whether there are any aspects of your dataset that limit your abilities to train and validate your model, and if so, understand why. In addition, when you are analyzing if your new model is any good or if you can expect to improve it any further, DQA allows you to leverage your dataset quality insights. And you get to do all that by measuring your dataset quality!
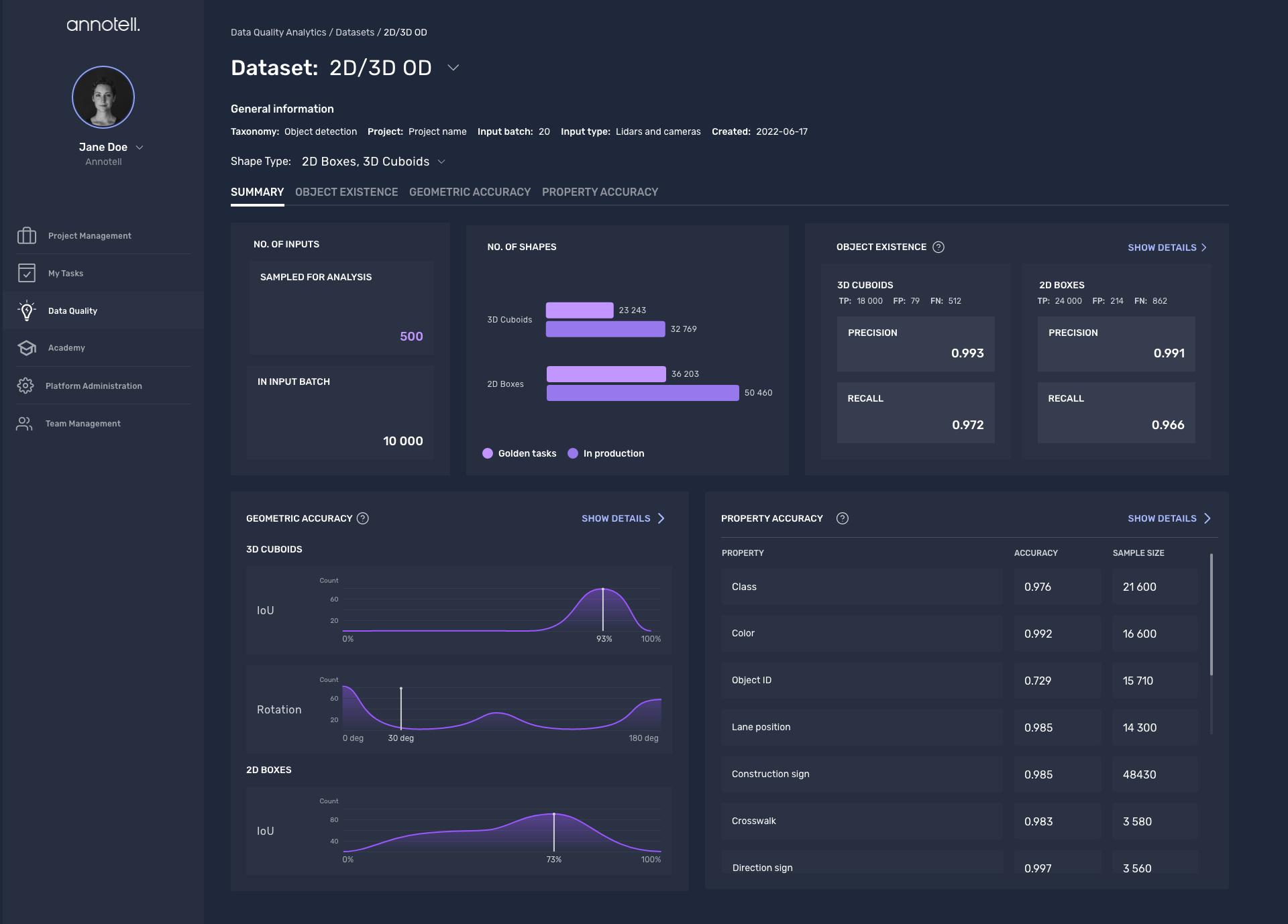
Let’s get practical
To illustrate this, let’s assume that you are working with a regression problem trying to predict the distance to an object or the length of it, and you are using the mean squared error (MSE) metric to validate your model. Even though you could obtain the perfect model regressing the variable of interest perfectly, the data is often somewhat noisy, which limits your capabilities to understand if you made an improvement with your last tweak or not. In the image below you can see a toy-example of this scenario. In spite of the fact that you had been able to perfectly train the model you would still see a non zero MSE value when validating. In this particular example, the low noise data yields a MSE value of 0.0090, whereas the high-noise data yields a MSE value of 0.160.
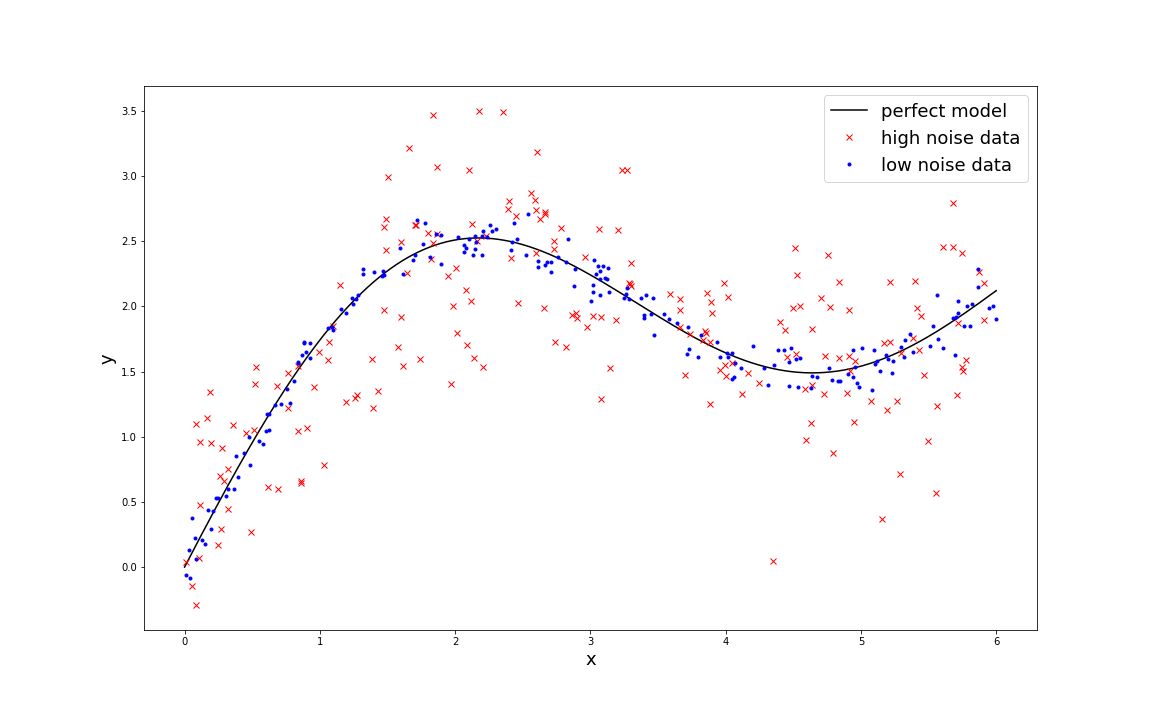
When using our dataset quality metrics, and by modeling the noise statistically and measuring the variability of the data, you are now able to infer your actual model performance. In this particular toy example, assuming normally distributed noise for the model and the data, it is straightforward to calculate your actual model performance: Variance(measured) = Variance(model) + Variance(data).
Here comes another example. Suppose that you are validating an object detection system and you measure an 85% detection rate compared to your test data. This value could be sufficiently good but you need to consider the detection rate of objects in your dataset as well. If your test dataset only contains 90% of all objects, your true system performance will only be 0.85 x 0.9 = 0.765, which is significantly lower than the metric you measured. To be able to claim that your model is any good you need to incorporate the dataset quality.
The real world is, of course, more complicated than that and requires sophisticated statistical models to enable you to draw the same conclusions as above, but the principle remains the same - you need to acknowledge your data variability to know your true performance and if the dataset quality is a limiting factor.
The efficient way to correctly interpret your model performance and draw informed conclusions
Dataset Quality Analytics will help you identify your true model performance and the upper limit on what you can expect to achieve. By doing so, you won’t have to spend time on trying to improve a model which you cannot measure an improvement for. Hello, efficiency 👋!
In other words, thanks to our dataset quality metrics you will be able to understand if the dataset quality limits your capabilities to develop a good system, as our product allows you to access our statistical data quality models which support all the common perception modalities. Thus, by using the Dataset Quality Analytics you will ensure that your models are exhaustively validated according to real world applications, increasing your engineering velocity.
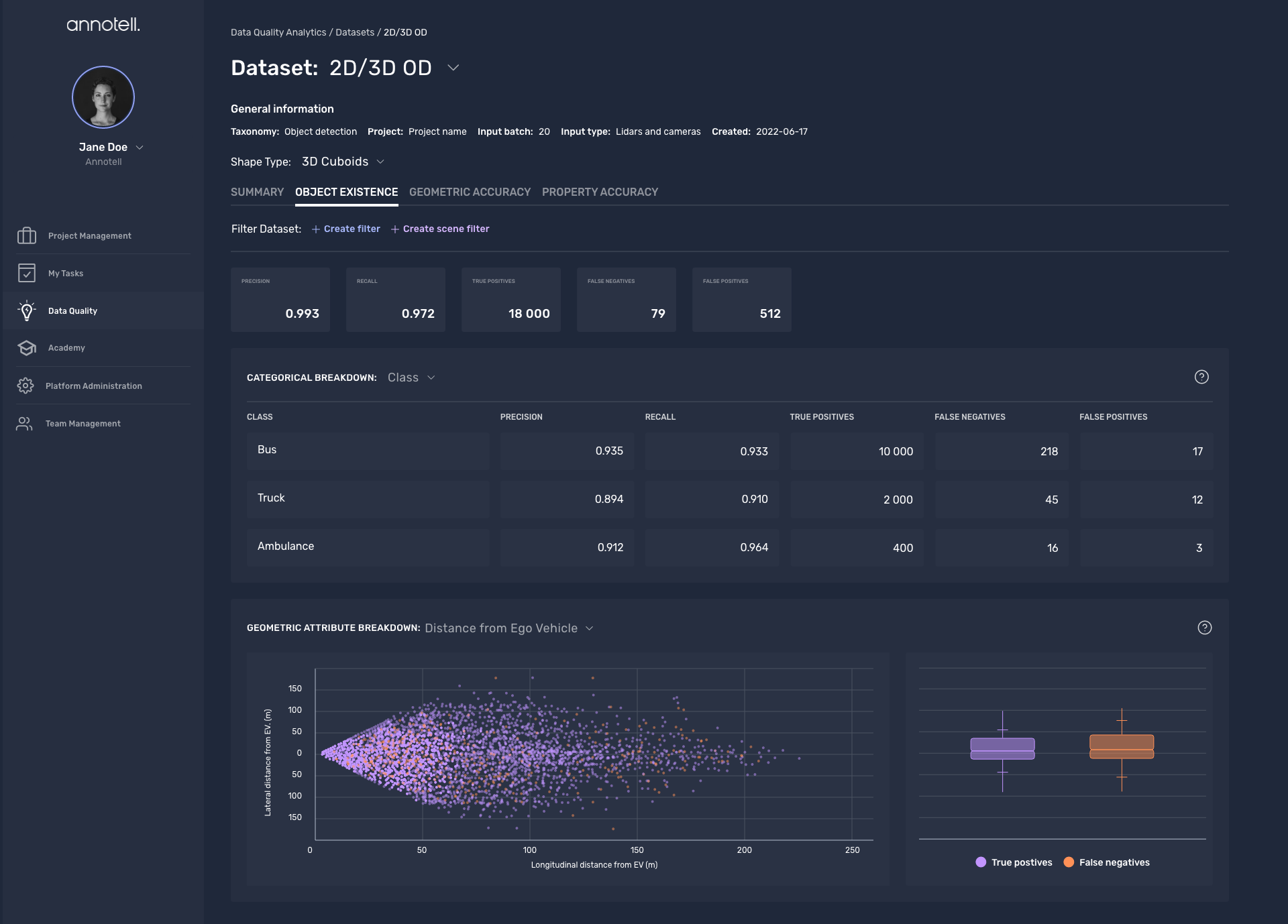
The question is: are you already empowering your team with this knowledge? We hear you, you cannot build safe perception in one day, and you need the right tools at your side. But once you provide your engineers with powerful tools, the magic will happen. But hey, we know it takes time. And we’re here to support you all the way. So feel free to reach out to us to discuss how you can unlock the value of our new Data Quality Analytics product and book a demo 🤗!
Share this
Written by
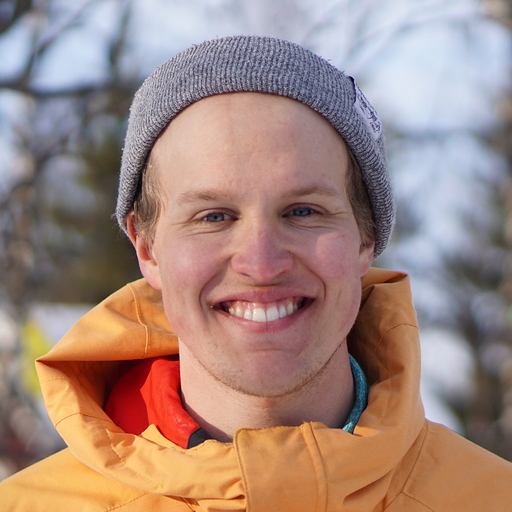
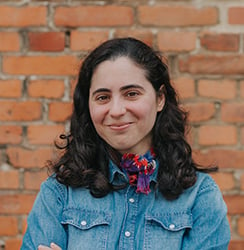